A Course in Machine Learning
by Hal Daumé III
Machine learning is the study of algorithms that learn from data and experience. It is applied in a vast variety of application areas, from medicine to advertising, from military to pedestrian. Any area in which you need to make sense of data is a potential consumer of machine learning.
CIML is a set of introductory materials that covers most major aspects of modern machine learning (supervised learning, unsupervised learning, large margin methods, probabilistic modeling, learning theory, etc.). It’s focus is on broad applications with a rigorous backbone. A subset can be used for an undergraduate course; a graduate course could probably cover the entire material and then some.
Support and Mailing Lists:
If you would like to be informed when new versions of CIML materials are released, please join the CIML mailing list. If you find errors in the book, please fill out a bug report. If you’re the first to submit an error, you’ll get listed in the acknowledgments!
Individual Chapters:
- Front Matter
- Decision Trees
- Limits of Learning
- Geometry and Nearest Neighbors
- The Perceptron
- Practical Issues
- Beyond Binary Classification
- Linear Models
- Bias and Fairness
- Probabilistic Modeling
- Neural Networks
- Kernel Methods
- Learning Theory
- Ensemble Methods
- Efficient Learning
- Unsupervised Learning
- Expectation Maximization
- Structured Prediction
- Imitation Learning
- Back Matter
Acknowledgments
Thanks to everyone who was ever a teacher or student of mine, to those who provided feedback on drafts, and to colleagues for encouragement to get this done! Special thanks to: TODO…
View Fullscreen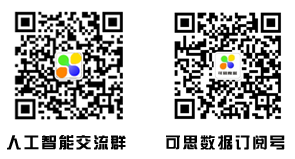
时间:2018-09-30 17:29 来源: 转发量:次
声明:本站部分作品是由网友自主投稿和发布、编辑整理上传,对此类作品本站仅提供交流平台,转载的目的在于传递更多信息及用于网络分享,并不代表本站赞同其观点和对其真实性负责,不为其版权负责。如果您发现网站上有侵犯您的知识产权的作品,请与我们取得联系,我们会及时修改或删除。
相关文章:
相关推荐:
网友评论: