Python-Cartopy包: 地理空间数据可视化
Cartopy 是为了向 Python 添加地图制图功能而开发的扩展库。该项目致力于以 matplotlib 包为基础,用简单直观的方式操作各类地理要素的成图。Cartopy 官网的画廊页面已经提供了很多绘图的例子,它们和官方文档一起,是学习该工具的主要材料。
本文亦提供一些例子,演示 Cartopy 在测量学等领域的应用,包括绘制中国政区图、IGS 站点分布、GNSS 控制网、地球板块分布、GNSS 速度场、电子含量分布以及突出显示某些地理要素等,旨在提供大地测量学方面的补充。
绘制中国政区图
本图使用兰伯特等积投影。Cartopy 默认使用的由 Natural Earth 提供的国界数据不符合我国的领土主张,本文的中国政区边界数据来自 GMT 中文社区,包含中国国界、省界、十段线以及南海诸岛。你也可以在 GMT 中文社区网站上下载单独的国界和十段线数据。在此向 GMT 中文社区的维护和贡献者表示感谢!
中国政区图
本图使用的中国国界、省界、十段线数据中,边界线数据块使用 “>” 号分隔。因此首先将其内容按照 “>” 切块,然后加载到 NumPy 中。绘图使用的代码如下:
import numpy as np
import matplotlib.pyplot as plt
import cartopy.crs as ccrs
import cartopy.feature as cfeature
# Load the border data, CN-border-La.dat is downloaded from
# https://gmt-china.org/data/CN-border-La.dat
with open('CN-border-La.dat') as src:
context = src.read()
blocks = [cnt for cnt in context.split('>') if len(cnt) > 0]
borders = [np.fromstring(block, dtype=float, sep=' ') for block in blocks]
# Set figure size
fig = plt.figure(figsize=[10, 8])
# Set projection and plot the main figure
ax = plt.axes(projection=ccrs.LambertConformal(central_latitude=90,
central_longitude=105))
# Add ocean, land, rivers and lakes
ax.add_feature(cfeature.OCEAN.with_scale('50m'))
ax.add_feature(cfeature.LAND.with_scale('50m'))
ax.add_feature(cfeature.RIVERS.with_scale('50m'))
ax.add_feature(cfeature.LAKES.with_scale('50m'))
# Plot border lines
for line in borders:
ax.plot(line[0::2], line[1::2], '-', lw=1, color='k',
transform=ccrs.Geodetic())
# Plot gridlines
ax.gridlines(linestyle='--')
# Set figure extent
ax.set_extent([80, 130, 13, 55])
# Plot South China Sea as a subfigure
sub_ax = fig.add_axes([0.741, 0.11, 0.14, 0.155],
projection=ccrs.LambertConformal(central_latitude=90,
central_longitude=115))
# Add ocean, land, rivers and lakes
sub_ax.add_feature(cfeature.OCEAN.with_scale('50m'))
sub_ax.add_feature(cfeature.LAND.with_scale('50m'))
sub_ax.add_feature(cfeature.RIVERS.with_scale('50m'))
sub_ax.add_feature(cfeature.LAKES.with_scale('50m'))
# Plot border lines
for line in borders:
sub_ax.plot(line[0::2], line[1::2], '-', lw=1, color='k',
transform=ccrs.Geodetic())
# Set figure extent
sub_ax.set_extent([105, 125, 0, 25])
# Show figure
plt.show()
绘制 IGS 站点分布图
本图使用的 IGS 核心站与 MGEX 项目站点,及其坐标均来自 IGS 网站。我已经将其整理成为 igs-core 和 mgex 两个 CSV 文件,你可以直接下载。
IGS 核心站与 MGEX 站点分布图
import numpy as np
import matplotlib.pyplot as plt
import cartopy.crs as ccrs
import cartopy.feature as cfeature
# Load the coordinate of IGS Core & MGEX sites, The CSV files are
# exported from: http://www.igs.org/network
igs_core = np.recfromcsv('igs-core.csv', names=True, encoding='utf-8')
mgex = np.recfromcsv('mgex.csv', names=True, encoding='utf-8')
fig = plt.figure(figsize=[9, 6])
# Set projection
ax = plt.axes(projection=ccrs.Robinson())
# Add ocean and land
ax.add_feature(cfeature.LAND)
ax.add_feature(cfeature.OCEAN)
# Add MGEX & IGS core sites
ax.plot(mgex['longitude'], mgex['latitude'], 'o', color='tomato',
label='MGEX', transform=ccrs.Geodetic())
ax.plot(igs_core['longitude'], igs_core['latitude'], '*', color='darkmagenta',
label='IGS Core', transform=ccrs.Geodetic())
# Plot gridlines
ax.gridlines(linestyle='--')
# Set figure extent
ax.set_global()
# Set legend location
plt.legend(loc='lower right')
# Show figure
plt.show()
绘制 GNSS 控制网
这里使用的 IGS 站点坐标数据同样来自 IGS 网站。我已将其整理成一个 CSV 格式的文件:euro-igs,你可以直接下载使用。这里使用 matplotlib.tri 中的 Triangulation 来根据输入的点位坐标来创建 Delaunay 三角网,然后使用 plt.triplot() 方法绘制。
GNSS 控制网
import numpy as np
import matplotlib.pyplot as plt
import matplotlib.tri as tri
import cartopy.crs as ccrs
import cartopy.feature as cfeature
# Load coordinate of the IGS sites in Europe, this CSV file is
# exported from: http://www.igs.org/network
igs_sites = np.recfromcsv('euro-igs.csv', names=True, encoding='utf-8')
# Generate Delaunay triangles
triangles = tri.Triangulation(igs_sites['longitude'], igs_sites['latitude'])
fig = plt.figure(figsize=[6, 8])
# Set projection
ax = plt.axes(projection=ccrs.LambertConformal(central_latitude=90,
central_longitude=10))
# Add ocean, land, rivers and lakes
ax.add_feature(cfeature.OCEAN.with_scale('50m'))
ax.add_feature(cfeature.LAND.with_scale('50m'))
ax.add_feature(cfeature.RIVERS.with_scale('50m'))
ax.add_feature(cfeature.LAKES.with_scale('50m'))
# Plot triangles
plt.triplot(triangles, transform=ccrs.Geodetic(), marker='o', linestyle='-')
# Plot gridlines
ax.gridlines(linestyle='--')
# Set figure extent
ax.set_extent([-10, 30, 30, 73])
# Show figure
plt.show()
绘制板块分布图
板块构造理论将地球的岩石圈分为十数个大小不等的板块。本图使用的 Nuvel 板块边界数据来自 EarthByte 网站,我已经将其整理为一个压缩文件,你可以直接下载使用。
Nuvel 板块分布图
import numpy as np
import matplotlib.pyplot as plt
import cartopy.crs as ccrs
# The plate boundary files
files = ['African.txt', 'Antarctic.txt', 'Arabian.txt', 'Australian.txt',
'Caribbean.txt', 'Cocos.txt', 'Eurasian.txt', 'Indian.txt', 'Juan.txt',
'Nazca.txt', 'North_Am.txt', 'Pacific.txt', 'Philippine.txt',
'Scotia.txt', 'South_Am.txt']
# Read boundaries into numpy
borders = []
for f in files:
border = np.genfromtxt(f, names=['lon', 'lat'], dtype=float, comments=':')
borders.append(border)
# Plate names
plates = ['African', 'Antarctic', 'Arabian', 'Australian', 'Caribbean', 'Cocos',
'Eurasian', 'Indian', 'Juan', 'Nazca', ' North\nAmerican', 'Pacific',
'Philippine', 'Scotia', ' South\nAmerican']
# Central point for every plate, just for text positioning
central = [(17, -5), (90, -80), (40, 21), (120, -28), (270, 12), (260, 6),
(60, 50), (70, 13), (230, 45), (260, -21), (250, 36), (190, 0),
(123, 17), (304, -59), (315, -27)]
# Start plot
fig = plt.figure(figsize=(12, 7))
ax = plt.axes(projection=ccrs.Mollweide(central_longitude=120))
# Plot a image as background
ax.stock_img()
# Plot boundaries
for plate, center, border in zip(plates, central, borders):
ax.plot(border['lon'], border['lat'], color='coral',
transform=ccrs.Geodetic())
ax.text(center[0], center[1], plate, transform=ccrs.Geodetic())
plt.show()
绘制 GNSS 速度场
本图使用美国西部的 GNSS 测站速度场数据,数据来自加州大学圣迭戈分校。在使用 ax.quiver() 方法绘制站速度矢量时,由于 matplotlib 自动确定的矢量长度比较短,不能够表现足够的细节。因此使用 scale_units 属性指定矢量长度单位,然后使用 scale 设置缩放程度。加州西部是著名的圣安德列斯断层,从下图中可以看出其两侧迥异的地质变化。
美国西部 GNSS 速度场
import numpy as np
import matplotlib.pyplot as plt
import cartopy.crs as ccrs
import cartopy.feature as cfeature
# Download the GPS velocity field from
# https://topex.ucsd.edu/CGM/01_GPS/wus_gps_final_names.dat
velo = np.genfromtxt('wus_gps_final_names.dat', usecols=range(1, 5),
names=('lat', 'lon', 'Vn', 'Ve'), skip_header=1)
# Coordinates of Los Angeles & San Francisco
l_a, s_f = (-118.30, 34.12), (-122.43, 37.74)
fig = plt.figure(figsize=(12, 12))
# Set projection
ax = plt.axes(projection=ccrs.Miller(central_longitude=-120))
# Add ocean, land, rivers, lakes & boundary of states
ax.add_feature(cfeature.OCEAN.with_scale('50m'))
ax.add_feature(cfeature.LAND.with_scale('50m'))
ax.add_feature(cfeature.RIVERS.with_scale('50m'))
ax.add_feature(cfeature.LAKES.with_scale('50m'))
ax.add_feature(cfeature.STATES.with_scale('50m'))
# Plot quivers
qs = ax.quiver(velo['lon'], velo['lat'], velo['Ve'], velo['Vn'], width=0.0015,
scale_units='inches', scale=85, color='darkred',
transform=ccrs.PlateCarree())
# Plot a legend for quiver
ax.quiverkey(qs, 0.04, 0.1, 20, '20 mm/yr', labelpos='E')
# Plot Los Angeles & San Francisco, as reference
ax.scatter(s_f[0], s_f[1], s=90, label='San Francisco', color='darkcyan',
transform=ccrs.Geodetic())
ax.scatter(l_a[0], l_a[1], s=210, label='Los Angeles', color='olivedrab',
transform=ccrs.Geodetic())
ax.legend(loc='lower left')
# Plot grid lines
ax.gridlines(linestyle='--')
ax.set_extent([-126, -113, 32, 40])
plt.show()
绘制电子含量分布图
Cartopy 以 matplotlib 包作为基础,可以使用 matplotlib 中的方法来绘制等值线图,只需在绘制时使用 Cartopy 处理地图投影变形。这里以绘制全球电离层电子含量图为例,模型来自北京航空航天大学·前沿电离层实验室,但只截取其产品文件 whug1420.18i 中第一个时段的数据,即使用的 1st-tecmap.dat 文件。
全球电子含量分布图
import numpy as np
import matplotlib.pyplot as plt
import cartopy.crs as ccrs
# Read VTEC data from a file
with open('1st-tecmap.dat') as src:
data = [line for line in src if not line.endswith('LAT/LON1/LON2/DLON/H\n')]
tec = np.fromstring(''.join(data), dtype=float, sep=' ')
# Generate a geodetic grid
nlats, nlons = 71, 73
lats = np.linspace(-87.5, 87.5, nlats)
lons = np.linspace(-180, 180, nlons)
lons, lats = np.meshgrid(lons, lats)
tec.shape = nlats, nlons
fig = plt.figure(figsize=(8, 3))
# Set projection
ax = plt.axes(projection=ccrs.PlateCarree())
# Plot contour
cp = plt.contourf(lons, lats, tec, transform=ccrs.PlateCarree(), cmap='jet')
ax.coastlines()
# Add a color bar
plt.colorbar(cp)
# Set figure extent & ticks
ax.set_extent([-180, 180, -87.5, 87.5])
ax.set_xticks([-180, -150, -120, -90, -60, -30, 0, 30, 60, 90, 120, 150, 180])
ax.set_yticks([-80, -60, -40, -20, 0, 20, 40, 60, 80])
plt.show()
突出显示某区域
Cartopy 默认对所有地物使用相同的外观,如果需要突出显示某些地物,就必须进行筛选。这里从 Natural Earth 提供的小比例尺国界数据中,提取出欧盟国家,然后使用 ax.add_geometries() 方法将它们加入到绘图元素中。
欧洲联盟
import matplotlib.pyplot as plt
import cartopy.crs as ccrs
import cartopy.feature as cfeature
import cartopy.io.shapereader as shpreader
# Country names in Europe Union, exported from Wikipedia:
# https://en.wikipedia.org/wiki/European_Union
eu_country_names = ('Austria', 'Belgium', 'Bulgaria', 'Croatia', 'Cyprus',
'Czechia', 'Denmark', 'Estonia', 'Finland', 'France',
'Germany', 'Greece', 'Hungary', 'Ireland', 'Italy',
'Latvia', 'Lithuania', 'Luxembourg', 'Malta',
'Netherlands', 'Poland', 'Portugal', 'Romania', 'Slovakia',
'Slovenia', 'Spain', 'Sweden', 'United Kingdom')
# Create a .shp file reader
shp_file = shpreader.natural_earth(resolution='110m', category='cultural',
name='admin_0_countries')
shp_reader = shpreader.Reader(shp_file)
# Reader the shape file
records = shp_reader.records()
# Collect the European Union countries
eu_countries = []
for rec in records:
if rec.attributes['NAME'] in eu_country_names:
eu_countries.append(rec)
# Start ploting
fig = plt.figure(figsize=[6, 6])
# Set projection
ax = plt.axes(projection=ccrs.Orthographic(central_latitude=50,
central_longitude=10))
# Add land
ax.add_feature(cfeature.LAND, facecolor='lightgray')
# Plot the European Union countries
for country in eu_countries:
ax.add_geometries([country.geometry], crs=ccrs.PlateCarree(),
facecolor='darkgreen')
# Plot gridlines
ax.gridlines(linestyle='--')
# Show figure
plt.show()
声明:文章收集于网络,版权归原作者所有,为传播信息而发,如有侵权,请联系小编删除,谢谢!
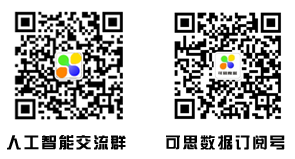
时间:2020-04-08 14:35 来源: 转发量:次
声明:本站部分作品是由网友自主投稿和发布、编辑整理上传,对此类作品本站仅提供交流平台,转载的目的在于传递更多信息及用于网络分享,并不代表本站赞同其观点和对其真实性负责,不为其版权负责。如果您发现网站上有侵犯您的知识产权的作品,请与我们取得联系,我们会及时修改或删除。
相关文章:
相关推荐:
网友评论:
最新文章
热门文章